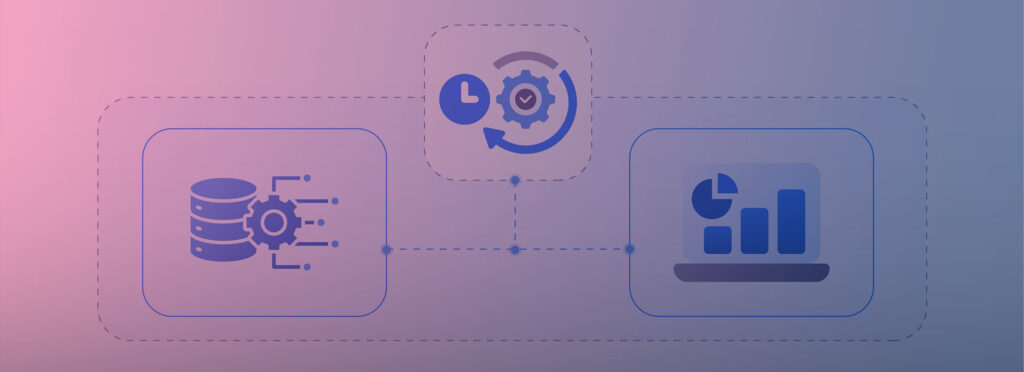
Real-time data powers instant decision-making across industries. This blog unpacks what it is, why it matters, and how brands like Shein use it to lead the market.
“At many of these companies today, including, I suspect, Shein, it’s not the fashion experts designing clothes,” says Lu, a researcher at the University of Delaware, in an insightful Rest of World article. “It’s engineers—engineers analyzing data.”
This observation underscores a paradigm shift in the fashion industry, epitomized by Shein’s meteoric rise.
The Chinese ultra-fast-fashion brand has disrupted the global market with a model powered not by traditional design expertise but by cutting-edge data analytics.
A Brief History of Fashion’s Evolution
In the 1960s and 1970s, haute couture brands like Gucci and Prada reigned supreme, defining global fashion with exclusive, high-end designs. By the 1990s, disruptors like Zara and H&M introduced the concept of fast fashion.
They slashed the runway-to-store timeline from six months to just three weeks, democratizing style with affordable, trend-driven clothing.
Fast fashion became faster still with the arrival of online-first brands like Fashion Nova, Boohoo, and ASOS.
These companies eliminated the need for physical stores and middlemen, leveraging the internet to streamline production and distribution. This evolution paved the way for ultra-fast fashion.
Enter Shein.
Shein by the Numbers
For those unfamiliar, Shein is a China-based ultra-fast-fashion powerhouse. Its staggering growth is reflected in these figures:
- $32.2 billion in global sales in 2023.
- 46.9 million unique visitors in the US alone by mid-2024.
- An 18% share of the global fast-fashion market, securing its category leadership.
- 25% of US consumers reported shopping at Shein in the past year.
- A global workforce exceeding 16,000 employees.
The Shein Model: Data Over Design
While predecessors like Zara focused on mimicking high-end designs with modest materials, Shein operates on a different principle altogether: data-driven agility.
Shein’s proprietary software continuously scans the internet—platforms like TikTok, Google Trends, and broader web ecosystems—for emerging trends. These insights feed directly into its manufacturing pipeline, enabling rapid iteration.
Using a lean methodology, Shein initially tests 10-15 designs, producing only a small batch. If a design resonates, production scales to thousands within days. The result? A feedback loop so fast that it cuts the design-to-market timeline to as little as three days.
Unlike traditional fashion houses, Shein doesn’t impose a singular aesthetic or brand identity. It has no distinct “style.” Instead, it acts as a real-time mirror of global trends, adapting its offerings to each market based purely on data.
Why Real-Time Data Matters More Than Ever
Every second, vast volumes of data are generated across the digital landscape—stock market fluctuations, viral social media posts, and shifts in consumer behavior occur at an unprecedented pace.
Real-time data processing has become a fundamental requirement for businesses aiming to thrive and outperform in competitive environments.
Batch processing, traditionally used to collect and analyze data in segments, often results in latency, making it unsuitable for applications requiring immediate action.
In contrast, real-time data processing eliminates delays by continuously ingesting, transforming, and analyzing data streams as they are generated, providing actionable insights in milliseconds.
Consider critical use cases: financial markets rely on microsecond-level data feeds to adjust trading strategies dynamically.
Fraud detection systems leverage streaming data to identify anomalies and prevent fraudulent transactions in real time.
Similarly, social media analytics platforms process millions of interactions per second to track emerging trends and user sentiment as they develop.
The stakes are high—time lags in processing can lead to missed opportunities, heightened risks, or delayed responses. Real-time processing ensures that data streams flow seamlessly, with systems adapting instantaneously to new inputs without defined beginnings or endpoints.
With the rise of Big Data architectures and the proliferation of diverse external data sources, the demand for real-time web scraping has surged.
Modern analytics engines—powered by frameworks like Apache Kafka, Flink, or Spark Streaming—thrive on continuous streams of fresh, structured web data to optimize AI-driven systems and accelerate decision-making pipelines.
At Grepsr, we specialize in delivering real-time web data tailored to your needs.
Whether enabling top-tier consulting firms to combat fraud or empowering global leaders in location analytics to outpace competitors, we ensure a steady flow of actionable data, precisely when it’s needed, to keep your business at the forefront.
Batch Processing vs. Real-Time Data Processing
In data integration, the Extract, Transform, Load (ETL) process has historically followed a scheduled framework.
Data is collected from sources such as APIs, databases, or flat files at specific intervals, transformed into a usable format, and then loaded into repositories like data marts or data lakes for analysis. This scheduled approach forms the foundation of batch processing.
The batch processing model emerged during a time when computing and storage resources were limited.
Processing large volumes of data continuously was infeasible, making batch processing the standard for tasks that didn’t require real-time insights.
Even today, it remains a reliable solution for use cases like generating periodic reports, running large-scale data migrations, or processing end-of-day financial transactions.
In contrast, the real-time ETL model processes data continuously, with minimal to no latency, enabling businesses to act on data as it is created.
This approach is particularly valuable in dynamic and fast-paced environments like retail, financial services, and telecommunications, where timely insights can significantly impact outcomes.
Consider Shein’s ultra-fast-fashion model. Using real-time ETL, Shein collects data from platforms like TikTok, Google Trends, and customer reviews to identify emerging trends.
If a spike in interest is detected—say, a specific dress style going viral on social media—Shein’s system immediately transforms this data into actionable insights.
Manufacturers are notified to create small test batches of the trending product within hours, not days or weeks.
If the product performs well, Shein rapidly scales up production, adjusting inventory levels in real time based on regional demand and customer feedback.
This seamless integration of real-time data enables Shein to avoid overproduction, maintain lean inventory levels, and stay ahead of their competitors.
Where Web Scraping Fits In
Real-time data is a game-changer, but accessing the right data at the right time isn’t always straightforward.
This is where web scraping steps in.
By automating the collection of information from websites, web scraping helps businesses gather external data—whether it’s about market trends, competitor activities, or customer sentiment—to power their decision-making.
Now, let’s address a key point: real-time is relative. Not every business needs second-by-second updates. For some, collecting data once a day is plenty. For example, a retailer might check competitors’ prices daily to adjust their own, while a social media agency may want updates on trending hashtags every hour.
That said, when real-time data is critical—like monitoring stock prices or detecting fraudulent activity—it comes with added complexity and cost.
Real-time scraping requires advanced infrastructure, which isn’t always necessary or worth the expense. The trick is finding the balance between how often you need data and the value it brings.
When Web Scraping Makes Sense
Here are some common use cases where web scraping—whether real-time or scheduled—can make an impact:
- E-Commerce: Track competitor pricing, stock availability, and customer reviews to refine pricing strategies and product offerings.
- Social Media Monitoring: Analyze trending topics, hashtags, or brand mentions to adapt marketing campaigns on the fly.
- Financial Services: Monitor market trends, sentiment, or commodity prices to make informed trading decisions.
- Retail Inventory Management: Understand product demand across different platforms to optimize inventory and logistics.
- Healthcare Insights: Gather updates on emerging health trends or pharmaceutical developments to stay ahead.
- Real Estate: Keep tabs on property listings, pricing trends, and competitor activity to remain competitive.
How Grepsr Fits Into the Picture
If collecting external data feels overwhelming, that’s where we come in. At Grepsr, we simplify the process by delivering exactly the data you need, when you need it—whether that’s in real time or on a regular schedule.
We handle the technical side, like navigating complex websites, dealing with CAPTCHA, and ensuring your data is delivered in a format that fits seamlessly into your workflows.
Plus, we make sure everything stays compliant and ethical, so you can focus on using the insights instead of worrying about the details.
Bringing It All Together
Real-time data processing isn’t just about speed; it’s about being smart with your resources and aligning data collection with your goals.
Shein’s example shows how quickly adapting to market trends can define success. And while not every business needs to operate at that speed, having the right data at the right time can still make all the difference.
Whether you’re tracking a fast-changing market or just staying ahead of competitors, web scraping is a powerful tool for developing a winning strategy.
With Grepsr, you get more than just data—you get the insights to keep your business moving forward.